Project C02: Interactive learning of explainable, situation-adapted decision models
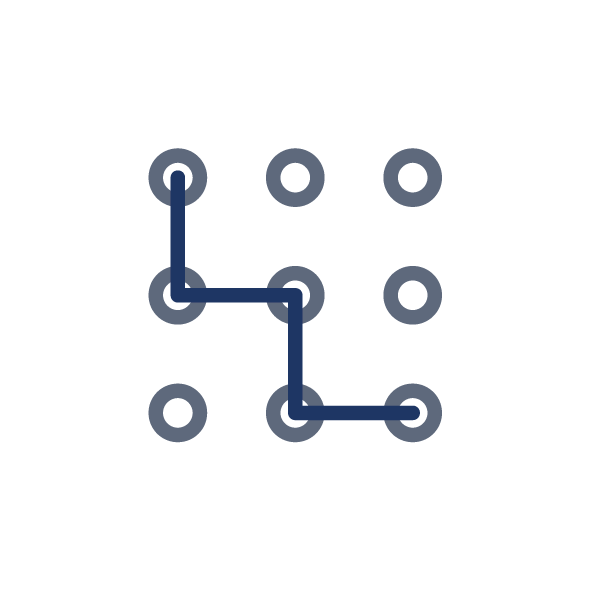
Different strategies are necessary for different situations in machine-based decision-making. The strategy to be used depends, for instance, on the amount of time or information available to make the decision. In Project C02, researchers from the fields of computer science and economics are working on a method to adapt decision-making models to different situations in which experts and users are incorporated in the process of construction. The goal is to enable decision-makers to choose the most suitable model and to be able to retroactively check the decision made.
Research areas: Computer science, Economics
Support staff
Nils Bojack, Paderborn University
Julia Rustemeier, Paderborn University
Luca Manuel Siekermann, Paderborn University
Publications
A. Lebedeva, J. Kornowicz, O. Lammert, J. Papenkordt, in: Artificial Intelligence in HCI, 2023.
J. Kornowicz, K. Thommes, Artificial Intelligence in HCI (2023).
J. Hanselle, J. Kornowicz, S. Heid, K. Thommes, E. Hüllermeier, in: Lernen, Wissen, Daten, Analysen (LWDA) Conference Proceedings, 2023.
J.M. Hanselle, J. Fürnkranz, E. Hüllermeier, in: Discovery Science, Springer Nature Switzerland, Cham, 2023.
S. Heid, J.M. Hanselle, J. Fürnkranz, E. Hüllermeier, International Journal of Approximate Reasoning 171 (2024).
M. Booshehri, H. Buschmeier, P. Cimiano, S. Kopp, J. Kornowicz, O. Lammert, M. Matarese, D. Mindlin, A.S. Robrecht, A.-L. Vollmer, P. Wagner, B. Wrede, in: Proceedings of the 2024 Workshop on Explainability Engineering, ACM, 2024, pp. 20–25.
Show all publications